Seeing beyond the scan in neuroimaging
Welcome to Practical AI, the podcast that makes artificial intelligence practical, productive, and accessible to all. If you like this show, you will love the changelog. It's news on Mondays, deep technical interviews on Wednesdays, and on Fridays, an awesome talk show for your weekend enjoyment. Find us by searching for the changelog wherever you get your podcasts. Thanks to our partners at fly.io.
Jerod:Launch your AI apps in five minutes or less. Learn how at fly.io.
Daniel:Welcome to another episode of the Practical AI Podcast. This is Daniel Witenack. I'm CEO at Prediction Guard, and I'm joined as always by my cohost, Chris Benson, who is a principal AI research engineer at Lockheed Martin. How are you doing, Chris?
Chris:Hey, I'm doing very well today. How's it going, Daniel?
Daniel:It's going great. I'm excited to kind of switch it up from all of the talk of language models and agents, although maybe that will feature somewhere in the conversation today, but turn to something that's really exciting and I'm really happy that we can feature on the show, which is all about neuroimaging and machine learning and AI, which will be an exciting topic to learn about. Today, we have with us Gavin Winston, who is professor at Queen's University. Welcome, Gavin.
Gavin:Good afternoon, thank you for the invite, Daniel.
Daniel:Yeah, yeah. Well, it's great to have you with us. Maybe if we can kind of backtrack from even the machine learning AI side of neuroimaging, even just to the context of neuroimaging, a lot of our audience might be in the technology space or software developers or business leaders. Could you help us understand a little bit When we're talking about neuroimaging, we're talking about some of the work that you're involved with, what does that mean exactly? How does it impact people's lives and the healthcare system and the treatments, etcetera?
Gavin:So neuroimaging is a pretty broad term. It covers a variety of different techniques. And essentially the general concept of these approaches is that we're looking at the structure and function of the brain. You can look at other parts of the nervous system as well, but for my work, I'm particularly interested in the brain. So for things like structure, can look at perhaps is there an abnormality within the brain that's causing someone seizures or other types of neurological problems?
Gavin:And on function, you can look at which parts of the brain are responsible for different functions that people have. For example, which parts of the brain are people using for language, which parts of the brain are people using for memory and other tasks like that. So I guess I'm more concentrating on MRI, but there's a whole variety of techniques. So things like CT scans and MRI scans and PET scans. There's a whole series of different things.
Gavin:But essentially they're just techniques to look into the brain from the outside try and give us an idea of the structure and function and how these might be altered. So I guess the most common thing people would see would be MRI scans. That's the thing, which is what I mainly work on.
Daniel:And maybe also give a little bit of context for some of the, I guess, more of a historical context for maybe when and how machine learning or AI techniques started coming into an intersection neuroimaging or MRI? How recent has that been? Is that something that's been going on for quite some time? And yeah, maybe just give a little bit of context there as well.
Gavin:I think maybe we can step back and think about how neuroimaging has developed over many decades.
Daniel:That'd be great. Yeah.
Gavin:So of course, back at the beginning, you first developed the concept of doing x rays and we could do an x-ray of the skull, But that wasn't particularly helpful because you couldn't see the brain inside the skull. You could just see the skull itself. So then people started developing other pretty barbaric techniques where you would inject air or other things into the brain and then you could somehow highlight them that that was pretty risky and certainly not that helpful. But I guess it started really coming in the 1970s when CT scans started to become available. So you could then get a nice illustration of brain or other structures from the outside.
Gavin:And then that developed further to MRI, which gives us much more detailed pictures looking at the brain. We can get higher and higher resolution and much more detailed now. As these techniques have developed, of course, we've got more and more data to analyze and the more and more data we have to analyze, that's when we start thinking, how can we use techniques such as machine learning to learn from this vast amount of data we're now starting to collect. So when you think about an MRI scan, you could have a resolution of two fifty six by two fifty six by two fifty six voxels. So it's a three-dimensional picture.
Gavin:But then you have multiple different types of MRI scans looking at different types of things within the brain. So you have an absolute ton of data. And when someone who is a radiologist, in those neurologists, sorry, a physician specialized in assessment of scans, they have a lot of information to look at. And that's extremely time consuming. And the number of scans we're doing is going up and up.
Gavin:So now we're starting to think, well, how can we help and sort of save time and also make it more easy to detect the abnormalities or automate things more effectively? Because the vast amount of data we have now, that's not possible for us to now keep up with.
Chris:I'm curious with the different types of scans and with all the data that they're producing and now that you have machine learning techniques available, has that changed some of the choices that doctors are making in terms of what's possible yet? Is that still more in the future? How has machine learning changed the practice of medicine in these areas?
Gavin:As with many things, when you're looking at the integration of machine learning and artificial intelligence into medical practice, the uptake of these techniques can be fairly slow. There's a fairly big chasm between what's possible technically versus what is actually used in practice. There's obviously a lot of concerns that people have around, data quality, ethics around using the data, the accuracy of any techniques you might be using because of course it's going to be used for humans that are undergoing different diagnoses and treatments. So there's a big gap between what can be done and what is actually being done in practice. So the uptake, I mean, there's a lot of potential there and you always see things being developed but the uptake has been a little bit slower than I would like as someone working in this field.
Gavin:But I think given what we can do now, if we think forward over what's gonna happen, definitely these are gonna become much more important over time.
Daniel:And maybe before we get into how a machine might process some of the data that you're talking about, if we just consider the human, the physician or whoever it is who's looking at some of the data coming off of these scans, I know there's probably a whole variety of things that could be discovered in that data in the imagery, right? But just by way of example, what might the human observer be looking for in the neuroimaging data that would give them a sense of, I guess it would be a diagnosis or something to investigate further or a potential abnormality or whatever that is? What are some of those things and what would they be looking for with their own human eyes?
Gavin:So when you are seeing a patient as a neurologist, will get a description of the symptoms and examine the patient as well. And that will give you an idea of where in the brain might be involved by whatever is going on. And then depending on the symptoms and the types of symptoms, you can get some idea of what types of abnormality could be occurring in that part of the brain. The role of the neuroimaging is really to confirm that there is an abnormality in that region of the brain and what it is. So where is it and what is the problem?
Gavin:Because you may have a list so called a differential diagnosis, you have a list of the possibilities it could be based on what you've managed to get from the patient. But, until you actually do the scan, you don't know exactly which one it is. So when a radiologist who's the physician looking at the scan, they will be provided the scan plus the clinical information and some hypothesis that the clinician is thinking about where it might be and what it might be. So then they're obviously going to look closely at those areas and try and identify something that correlates with that. And for a radiologist looking at things, lot of it is about pattern recognition and recognizing things that they've seen before.
Gavin:So sometimes it's very hard for a person to define what it is in the image that they see that tells them it's a certain thing, but it's, they've seen this before. And that's, that's what it is because there's a certain pattern that somehow they've learned that represents that.
Chris:Got a quick follow-up, you know, as Dan asked the question and you were answering it, it occurred to me how little I know about the topic as a layperson, obviously. And you kind of started with the notion of kind of two things that there was structure and there was function of the brain. And I'm kind of curious, could you take a second kind of backing away from the data side of things, but just kind of going into that and talk about, if you're a neurologist, what is the relationship between structure and function in the practice even before you get to the data? So how do they relate in terms of diagnosis and your evaluation of a patient? And how might that inform bringing data into it, as Dan brought up in that last question?
Gavin:So most of the scans that are done in day to day life would be scans looking solely at the structure of the brain. So for example, if someone has presented with symptoms that you think represent a stroke, but we want you to do a scan to work out is there a stroke and where is the stroke. So you're looking at the structure of the brain. So pretty much all the clinical scans out there being done are scans specifically looking at structure. So there's a whole separate side of imaging when we're talking about MRI imaging, which is looking at the function of the brain.
Gavin:And this is used in specialist centers and in specialist situations. So for example, if we're contemplating doing a surgical treatment on the brain to treat some underlying condition, of course, we don't want to know just what the brain looks like. We want to know which parts of the brain are performing different functions. We know in general that certain tasks are localized to particular parts of the brain but each person is individual and it may be slightly changed by the underlying abnormality they have. So there are certain scans you can do to look at the function.
Gavin:So for example, if you want to do some brain surgery near the visual pathways of the brain, you might want to identify where the visual pathways are by some form of functional imaging. Or if you're doing surgery near where language function may be, you want to know exactly where in the brain language function is so you can try and avoid that area if possible. So these are so called functional scans when you're looking at the function of the brain. But that's far, far less common in day to day practice.
Daniel:This may be an interesting question, but it's not often we have someone who's on the show that's both an expert in machine learning AI type of things and neuroscience or neuroimaging. I'm wondering, from over the years, of course, we've had many people on the show, there have been many parallels between neural networks and the structure of the brain and how these things are maybe modeled after one another. I'm wondering from a kind of expert in the field who's also applying machine learning and AI techniques, just how maybe complicated or different the brain might be than these kind of neural networks or deep learning systems that, yes, are very powerful, but at least in my understanding at their root contain very simplistic components and certainly aren't as efficient as the brain in many ways. I don't know if you have any thoughts on that, but I figured I would take the chance because we don't often have this intersection of expertise on the show.
Gavin:That's a great question. And I think, if you think about neural networks, as you mentioned, of course they are based on biology and what happens in reality, but there's quite a big difference between what we're simulating and what the reality is. And a lot of it is around the scale. So when we when we have neural networks, although now of course we can have much more complicated neural networks with the computational power we have now than we used to, you don't realize just how complicated the brain is, just how many billions of neurons it has and how they're all vastly interconnected. So that type of complexity has been very, very difficult to emulate and even when we try and emulate the nervous system of very simple organisms that only have a few hundred neurons, it's very difficult to replicate that precisely.
Gavin:So how can we possibly do the same for a structure like the human brain, which has so many more orders of magnitude neurons and synapses there? But yes, it's based some underlying anatomy and function within the brain. But there's a big gap between the level of complexity of what we simulate and what we actually are doing in our own brains.
Daniel:Gavin, now that we have a bit of context about neuroimaging and the brain in general, I'm wondering if you could give us just now zooming in a level down, I guess, or more focusing on the computational techniques. At kind of a high level, how would you categorize the ways in which machine learning or AI is being applied to tasks related to neuroimaging?
Gavin:There are a number of different ways that we're using machine learning applied to neuroimaging, tackling various different parts of a patient's diagnostic and treatment journey. So one example would be trying to classify scans as to whether they contain an abnormality or not. So that's a simple classification task. A lot of the literature out there, they collect data on some healthy individuals without the underlying condition. Then they also collect some data on some people with a particular condition.
Gavin:And the aim of the machine learning algorithm is to try and classify whether someone has a particular condition or not on the basis of the imaging. You can publish quite a lot of papers doing that, but the question is how useful is that in the real world? Because it's very unlikely you'll be saying, us wanting to ask it, do you have condition X or not, which is what essentially what the classifier is doing. You have a patient in front of you and you want to know what condition they have not do they have condition X or not. So therefore, you need to go a much, much higher level than that trying to classify amongst different abnormalities.
Gavin:Another way that, classification could be used is not just does a person have condition X or not, but it may be a condition that could be in various different parts of the brain. So what you're trying to classify is which part of the brain is affected by this condition, and which part of the brain is normal, healthy tissue. And that's particularly, something I do in my work in epilepsy. So epilepsy is a condition with recurrent seizures coming from the brain. And in some cases, those seizures may be coming from a particular part of the brain.
Gavin:We want to know where the abnormality in the brain is causing that's causing those seizures is located. Apart from that, what I've just mentioned about diagnosis, then another thing that's being used is how can we use imaging to help us guide what type of treatment someone should have, which is the best treatment given this scan we have, and also can we infer some information about the prognosis of a patient. If we treat them, what is the likelihood of a particular outcome? So for example, if brain tumor, for example, what's the likelihood of someone recovering from that or passing away from the underlying brain tumor? Can we predict that from our imaging data?
Gavin:So it's used for a whole variety of things from diagnostic purposes through to treatment options and prognosis as well.
Chris:So I'm curious that the use cases that you were kind of talking about applying data and machine learning techniques to were really fascinating to me. You know? And could you take as an example one or or or more of them and kind of talk about which ml techniques that maybe our listeners are familiar with, you know, that they've used in different, in their own jobs that are unrelated, and talk about, well, I take this and I apply it to, classifying what part of the brain is affected, by epilepsy. Kind of tie in a little bit about what a practitioner that is listening might have done themselves and they might never have occurred to them to use it in this way. I'm trying to kind of tie the technique with the application itself a little bit.
Chris:If you could kind of just take us through a couple of examples possibly on that.
Gavin:With the first example I gave of just trying to classify as whether someone has a disease or not. Typically, we extract a number of different parameters from the imaging and that could be designed in many different ways. And then we have a known output. So this is they either have the disease or not because we decide to scan people predefined disease or not. So this is essentially a supervised learning approach and you can just use typical techniques such as a support vector machine that you may have seen in many other approaches.
Gavin:This is a very common thing that is used in this type of approach. Then some more of the advanced imaging techniques when you're looking at the whole three-dimensional picture of the image, this is very well suited to techniques such as convolutional neural networks. They're extremely good at doing this type of imaging analysis. So I've just mentioned two techniques, but these are probably the most common two things you will see in the literature, but there are plenty of other options as well.
Daniel:And with that, I'm imagining that I've seen a lot of problems in supervised learning with I get a dataset and I train the model. I've seen computer vision techniques. I'm imagining there's a number of unique challenges on the technical side with what you're doing, which may be related to the data, maybe it's related to the scale, maybe it's related to the correlations, maybe it's related to the complexity of the number of target classes. Maybe just help us understand what are the challenging elements in any of those aspects as you apply those kind of general purpose techniques in this specific case?
Gavin:One of the biggest challenges is having access to the data in the first place. So, when you look at techniques such as large language models that have a vast trove of data on the internet that they can tap into, unfortunately, don't have the same thing when we're approaching neuroimaging. So if we want to train a model for whatever task we're trying to train it for, the more data we have in general, the better we can train the model. But the question is where do we get this data from? And that's one of the biggest challenges.
Gavin:So, if you are running a project at a university and you're scanning subjects for a research study, you may be able to get a hundred subjects. But a hundred subjects to someone in machine learning, that is a minuscule number. So that is nothing like hundreds of thousands of data points that you might want. So the first thing is getting the data. And there have been approaches to try and combine data from other centers and multicenter approaches and so on, but still we're limited by the amount of data.
Gavin:And of course, if you're using a supervised learning approach, the data has to be labeled as well. And depending on the nature of the label, that could be extremely laborious and time consuming and maybe inaccurate depending on who's doing that. If we're just classifying someone as having a condition, a disease or not, that's a pretty easy classification to make. But again, mistakes could be made. But if we're doing some of the more complex things, such as trying to work out which part of the brain is affected by a particular condition, then often the way this is done is by someone labeling the image.
Gavin:In other words, someone draws manually in the brain which part of the brain is affected, and that's what's used to train the algorithm. But given how I mentioned that there's so many voxels in the brain, the amount of time it takes someone manually to draw around the abnormality in the brain, that can be extremely time consuming, several hours per subject. So if you have to do that on a hundred subjects, that's a lot of manual work. So it's extremely difficult to get the data plus also the labels for the data. So that's a pretty big challenge for any clinically based study using machine learning and AI algorithms, just getting that in the first place.
Chris:Given the limitations of the available data, in this field, is there any role for synthetic data? In some other areas unrelated that is acceptable and in others I've heard reasons why it's not. Is any level of synthetic data that you're producing to support the research, is that a possibility? Is that something that you stay away from? Just curious.
Gavin:Sometimes people augment their data by synthesizing slightly modified versions of the data they have and use that for training. So that is something that can be done. But I don't think you can synthesize data completely from scratch. You can just modify some existing data you already have. Yes, that's certainly a possibility.
Daniel:And mostly you've talked about some of these approaches, convolutional neural nets, support vector machines. Again, I think a lot of the questions we're asking are coming from a place of those not working in the field and questions that people might have from outside of the medical field. A lot of what I've heard is that there is definitely more of a burden for explanation of certain predictions and a sort of audit trail potentially of how decisions were made. Obviously, that's easier potentially with a machine learning model than with a deep neural net. You know, what what is the reality there in terms of the techniques available to you?
Daniel:Not so much technically, but from but from a practicality of how they might be might be applied. I guess one thing is proving something in, in a paper, right? But then actual adoption of that could be challenging. So what are the realities there?
Gavin:Yeah, we already discussed that unfortunately implementation is well behind what it would ideally be at this stage. And I think one of the challenges is if you develop an algorithm and you present it to a physician and say, look, this does such and such. They want to understand how that's working. They want to be able to trust the algorithm. So if you've developed an algorithm that's meant to detect disease X, how is it making that decision?
Gavin:Is it doing something that makes sense to me? Because ultimately, of course, a lot of the AI algorithms could be just appeared to be like a black box. Don't you've got your input, you've got your output, but you don't really know what went on between those two steps. And particularly for physicians less familiar with these techniques, they want to know what's happening so they can trust the algorithm. Because at the end of the day, you're going to be making a decision that can affect someone's life on the basis of this information.
Gavin:So you want to be sure how that works. So certainly some of the imaging analysis programs are starting to work more towards the concept of explainable AI as you mentioned. So actually, I can mention one study here that's a study that I'm contributing data to, but it's led by my colleagues at UCL. It's their MELD study. It's a multicenter epilepsy lesion detection study.
Gavin:And this is a study which is meant to help us detect where in the brain is causing, epileptic seizures. And they're collecting data from many different sites across the world. And one of their key aims is to develop something that explains why the decision is being made. So the output of the algorithm is not only just this is where in the brain we think there may be an abnormality. There is also then an output that says these are the features that were different in that region of the brain that have led us to believe that that is where the abnormality is.
Gavin:And then when we look at that, we could potentially even ask a radiologist to go back and look at that part of the brain again and say, look, this is what we're seeing is potentially different. Are you able to now see that on the image?
Chris:I'm curious, a couple of points in the conversation, you've talked about kind of the speed of adoption of the technologies and you addressed a little bit about what some of those root causes are in terms of the desire to understand algorithms such as that. On behalf of the practitioner, the medical practitioner, culturally within the profession, what do you what kinds of mind shifts do you think are going to need to take place, maybe to accelerate adoption or what, you know, what is that natural progression that you're seeing? Because, as we're seeing these technologies flood into completely different industries across the globe and in different capacities, we're seeing these kind of cultural struggles within given professions on that. And I'm really curious as you you look at these doctors that are at some level adopting the technology and moving forward with it, recognizing the benefits, but also some of the challenges based on their traditional thinking. What do you think it'll take to get there for that profession?
Gavin:The use of AI in our day to day life has now become so widespread. I think people are becoming much more acceptable of the technology as a concept. But when we're working with clinical data, one of the limitations we have is what are the ethical considerations behind that? And that's one barrier to adoption. So where is the data that we've acquired from a patient going?
Gavin:Is it if we're submitting it to some algorithm, where is it being processed? Where is it being stored? Is it being kept? Is it being used for other things in the future? Is covered by privacy law, etc?
Gavin:So there's a lot of considerations there. But if we can get something which addresses all of those concerns, I think now is the time in the next decade and so on, where you can actually start to get these things much more widely adopted because there is that widespread acceptance of AI now.
Daniel:So Gavin, I'm wondering, obviously you've done a variety of research in this area and are aware of other things that are going on. We've mostly talked about kind of the context, the background of the problem, the technology, the challenges. I'm curious a little bit about the potential impact or performance. Let's say that you're doing one of these studies, maybe you could give an example. What is the comparison between a human maybe that's doing this sort of review manually and identifying either certain diagnoses or parts of the brain?
Daniel:I imagine things get more complicated as the problem gets more complicated. But what's kind of the human performance level and where have people been able to push the kind of machine learning AI performance level? Now, granted, as you mentioned, there's still challenges to overcome with the adoption, but I'm curious, at least in the studies that you've done or have seen, how is that stacking up? Maybe also, what does it seem like these models are really good at? And then maybe what are some of the open challenges that are not addressed currently?
Gavin:Yeah. So the performance of humans in addressing whether there's an abnormality on the scan is very, very rare. There are a lot of studies out there that look at inter rater performance between different people looking at the same type of data. And unfortunately, the performance and the indigreement can be quite poor in some cases. And if you look at trying to detect some subtle alteration in the brain, there are studies out there that show that the more expertise and the more highly trained the specialist is, the more likely they are to detect the abnormality.
Gavin:So if you have someone who's a highly specialized neuroradiologist only looking at scans of people with epilepsy, they are much more likely to detect it than any abnormality than someone who's a neuroradiologist looking at all sorts of scans. And they're in turn more likely to detect it than someone who's a general radiologist, not just specifically looking at neuroradiology. So there's a lot of, as with anything in life, the more highly specialized and trained you are for something, the more likely you are to detect things on a scan. Having said that, the reason we want to try and use AI sometimes is to look for things that aren't easily detectable by the human eye. There are certain things that are very hard to visually perceive, but there are patterns in the imaging data that can be picked up by the algorithms.
Gavin:Think that's a very strong case for the use of AI for things that really are not visually apparent or easy to detect. But we can use it in a lot of other, aspects of our life as well all through the whole process. So if we're putting in requests for scans, of course, there's going to be a waiting list for scans because many scans are being requested. Perhaps there's some way you can look at the information given on the patient and decide which ones are the more urgent, which ones are more likely to yield something abnormal that affects how I treat the patient. And then once you've done the scans, a radiologist will be given a list of scans to look at.
Gavin:But if you can pre assess those scans with some form of algorithm that prioritizes the scans and say, these five scans appear to have an abnormality. Look at these five scans first. That's much more useful. And then you can leave the other 100 scans that are probably normal to later you prioritize the ones that are potentially going to change someone's treatment. And then we could go on.
Gavin:There's just many steps in the whole process that you could integrate this into.
Chris:I'm curious as you're kind of defining kind of how it's changed the current workflow where the automation is the model is kind of pre selecting scans to the radiologists, But yet, with models advancing so fast right now in terms of the capabilities, would you expect that that to kind of remain the relationship between the model's capability and the human radiologist? Or do you think that that's going to evolve over time? And is that a constant evolution that you anticipate? Or do you think there's some sort of human AI steady state in terms of the the collaboration between the technology and the human that's going.
Gavin:Yeah. I guess, I mean, we're often asked is AI gonna replace the physician? Indeed. Essentially that type of question. I personally do not think it will, but I think it's going to be a technique that facilitates and helps.
Gavin:In other words, it's going to augment the abilities of whichever type of physician you are. It will make your workflow more efficient, more smooth and so on but it's never going to completely replace the human aspect. So for example, now when we use we use any of these techniques and identify things on imaging which we think may be relevant from the AI, we always then present it back to the team physicians to look at the scan again and then determine whether we think that's relevant or not. It's not replacing what we do. It's giving us some information which we then go back and review ourselves.
Gavin:So for example, there's something that may have been overlooked or very hard to see in the first place. An algorithm that says, possibly there's an abnormality here. You can then go back and confirm or refute whether you think that's the case or not. So it's essentially, I think it's going to remain this type of thing augmentation of what you do, but not a not a replacement.
Chris:So and and if I could just follow-up for a two second thing on that. And I'm not disagreeing with you, but I am curious because people will say, I think the human will stay in the loop and stuff in that. But why, what is in the way that you're looking at it, why do you think the human will stay in the loop? And I'm not arguing against that or saying that's a bad thing at all, but I've gotten that when I tell people that I think a human will stay in various workflows and other industries and stuff, that's a common question is they go, well, why? Based on what you're telling me, with the model increasing.
Chris:I'm kind of curious what your kind of foundational belief is there.
Gavin:Well, however good algorithms are, they do make mistakes. And one of the big issues in the type of algorithm I'm talking about, which is trying to detect where an abnormality in the brain is the cause of seizures, is a lot of false positives. So the technique looks good on the basis of that we are identifying where the abnormality is, but it doesn't address the fact that maybe three or four other brain regions that were also identified that were not involved at all. So you're getting a lot of false positives. So that performance of the algorithms is good, but far from perfect.
Gavin:So I think that's a key reason why you're always going to need some human oversight and looking into that. And of course, then there's a whole separate issue of what is the legal responsibility. If an algorithm says X and you make a decision based on that and it turns out what it said was wrong, whose responsibility is that? Is it the person who used the algorithm? Is it the person who wrote the algorithm?
Gavin:Is it the physician? Which physician is it? It's a difficult decision. So I think there's always going to have to be some form of human oversight in the process.
Daniel:And how on that front, on the legal side, are jurisdictions or governing bodies whatever the relevant kind of association would be, are those bodies keeping up with this sort of work and ahead and putting the legal frameworks in place? Are they catching up a combination of the two? What guidance is coming down and how does that legal situation look as of today?
Gavin:That's not something I've looked into a lot because each country has its own different rules. But in general, unfortunately, the legal system lags a lot behind the technological innovations that are occurring in the world. So there's definitely a lot of scope to working out all of these legal issues and how they best dealt with.
Daniel:Yeah. I know, speaking of things changing in the world, obviously we've seen a huge shift and perception shift and shift in technology and AI over the last couple of years with Gen AI and language models, vision models, and all sorts of things, I imagine that there is discussion in the research community around how these types of generative technologies might play a role in maybe it's the decision support around this type of work or other things. Is there any perspective you have there, or have you crossed paths with folks that are considering those sorts of techniques in addition to the machine learning, traditional machine learning models or CNNs or deep learning type of models? What's the status there in terms of reception or integration of this latest wave of technology?
Gavin:That's not an area I work in much myself as I mainly concentrate on the image analysis side of things. But I think that goes back to what I mentioned earlier about the potential triage opportunities of these types of approaches. So given this textual information on why we're doing a scan plus access to someone's medical records, what are the likely possibilities? What's the probability of these things actually being the case? Which is the most urgent scan that we should be doing next?
Gavin:I think I see it in that area is when it's going to be the most useful.
Chris:As you were looking at this, find it really fascinating. I'm of an age where I'm in my mid fifties and having lots of medical procedures and seeing the advancement of technology pretty rapidly. As you look at this area that you focused on so much, where do you envision the technology taking the profession and the tools of the profession? And how do you think that will will go forward in terms of as we have ever more AI capabilities and algorithmic capabilities and more data available, what does the future of imaging look like to you, and where do you think it might go, and there any particular things that you would like to see happen as you've as you've thought about your work and where it's going and you know, the the rate of adoption? I'm just curious what that future is.
Gavin:Yeah.
Gavin:What what I would like to see is as a radiologist sit stand to report 20 scans they've got, instead of scan one to 20, it's scan most likely to be abnormal to least likely to be abnormal. So you've already got your list of the order you should be looking at them. And when you open the scan, rather than just seeing the scan itself, just the scan, there's an algorithm been run on it already to perform the type of analysis you're interested in. And that's generated a report, some recommendations and ideas, has already been fed back into the radiology system. So when you open it, it's not just the scan, it's the scan plus sort of computer generated recommendations report.
Gavin:So you already have that information ahead of you so you can then focus on those areas and then potentially detect things that may have been overlooked before or get things more quickly. And then once the report has been done, then you need some way that that's going to be fed back to the treating physician in a useful manner. At the moment, what happens is the radiologist writes a report and that is sent electronically or sadly, in some cases by paper to the referring physician to look at when they get around to looking at it. But maybe there's some AI that can be put in at that stage which can generate a recommendation or prioritization of those information, those results so that the physician that actually requested the scan in the first place is alerted sooner when there's a significant abnormality that needs to be addressed.
Chris:Sounds good to me. Yeah, definitely.
Daniel:I think that's a great picture to paint as we close-up here. Gavin, it's been a great experience having you on the show. I encourage people in the show notes. We'll we'll include a couple links to where you can find some of Gavin's papers and and presentations. I encourage you to check it out.
Daniel:Really appreciate the work that you're doing Gavin and appreciate you taking time to chat with us. It's been great.
Gavin:Okay, great. Thank you very much.
Jerod:All right. That is our show for this week. If you haven't checked out our changelog newsletter, head to changelog.com/news. There you'll find 29 reasons. Yes.
Jerod:29 reasons why you should subscribe. I'll tell you reason number 17. You might actually start looking forward to Mondays. Sounds like somebody's got a case of the Mondays. 28 more reasons are waiting for you at changelog.com/news.
Jerod:Thanks again to our partners at Fly.io, to Brakemaster Cylinder for the Beats, and to you for listening. That is all for now, but we'll talk to
Jerod:you again next time.
Creators and Guests
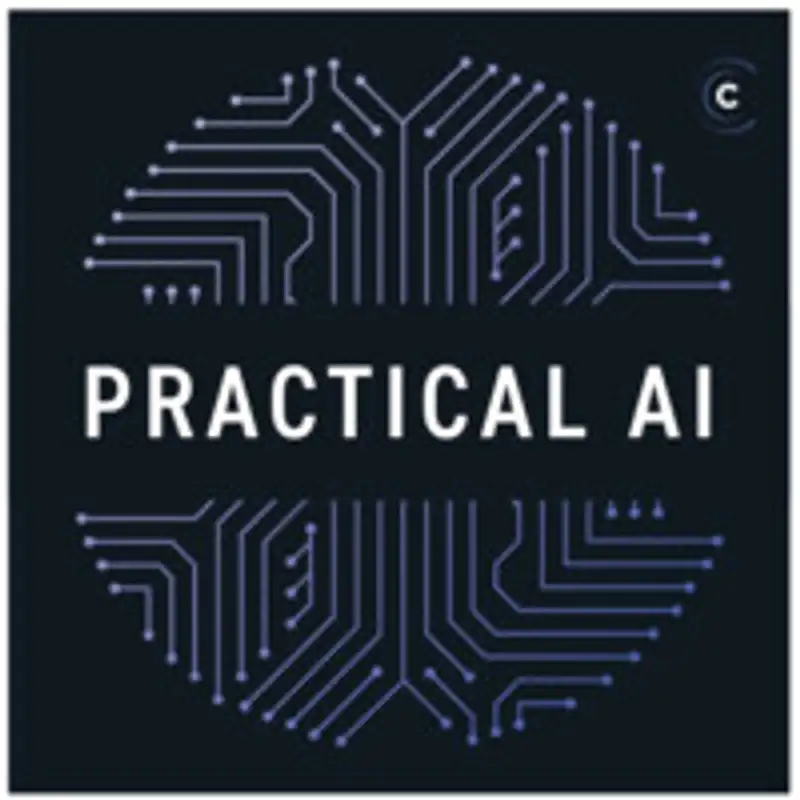